Machine Learning Framework PyTorch Enabling GPU-Accelerated Training on Apple Silicon Macs
In collaboration with the Metal engineering team at Apple, PyTorch today announced that its open source machine learning framework will soon support GPU-accelerated model training on Apple silicon Macs powered by M1, M1 Pro, M1 Max, or M1 Ultra chips.
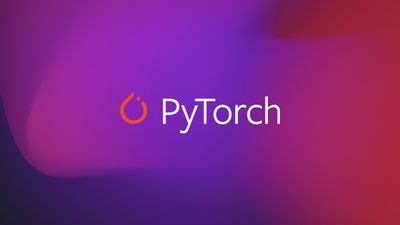
Until now, PyTorch training on the Mac only leveraged the CPU, but an upcoming version will allow developers and researchers to take advantage of the integrated GPU in Apple silicon chips for "significantly faster" model training.
A preview build of PyTorch version 1.12 with GPU-accelerated training is available for Apple silicon Macs running macOS 12.3 or later with a native version of Python. Performance comparisons and additional details are available on PyTorch's website.
Popular Stories
The long wait for an Apple Watch Ultra 3 appears to be nearly over, and it is rumored to feature both satellite connectivity and 5G support.
Apple Watch Ultra's existing Night Mode
In his latest Power On newsletter, Bloomberg's Mark Gurman said that the Apple Watch Ultra 3 is on track to launch this year with "significant" new features, including satellite connectivity, which would let you...
The iPhone 17 Pro Max will feature the biggest ever battery in an iPhone, according to the Weibo leaker known as "Instant Digital."
In a new post, the leaker listed the battery capacities of the iPhone 11 Pro Max through to the iPhone 16 Pro Max, and added that the iPhone 17 Pro Max will feature a battery capacity of 5,000mAh:
iPhone 11 Pro Max: 3,969mAh
iPhone 12 Pro Max: 3,687mAh...
Apple's next-generation iPhone 17 Pro and iPhone 17 Pro Max are just over two months away, and there are plenty of rumors about the devices.
Below, we recap key changes rumored for the iPhone 17 Pro models.
Latest Rumors
These rumors surfaced in June and July:Apple logo repositioned: Apple's logo may have a lower position on the back of the iPhone 17 Pro models, compared to previous...
The upcoming iPhone 17 Pro and iPhone 17 Pro Max are rumored to have a slightly different MagSafe magnet layout compared to existing iPhone models, and a leaked photo has offered a closer look at the supposed new design.
The leaker Majin Bu today shared a photo of alleged MagSafe magnet arrays for third-party iPhone 17 Pro cases. On existing iPhone models with MagSafe, the magnets form a...
Apple's position as the dominant force in the global true wireless stereo (TWS) earbud market is expected to continue through 2025, according to Counterpoint Research.
The forecast outlines a 3% year-over-year increase in global TWS unit shipments for 2025, signaling a transition from rapid growth to a more mature phase for the category. While Apple is set to remain the leading brand by...
iOS 26 and iPadOS 26 add a smaller yet useful Wi-Fi feature to iPhones and iPads.
As spotted by Creative Strategies analyst Max Weinbach, sign-in details for captive Wi-Fi networks are now synced across iPhones and iPads running iOS 26 and iPadOS 26. For example, while Weinbach was staying at a Hilton hotel, his iPhone prompted him to fill in Wi-Fi details from his iPad that was already...
Apple today seeded the second betas of upcoming iOS 18.6 and iPadOS 18.6 updates to public beta testers, with the betas coming just a day after Apple provided the betas to developers. Apple has also released a second beta of macOS Sequoia 15.6.
Testers who have signed up for beta updates through Apple's beta site can download iOS 18.6 and iPadOS 18.6 from the Settings app on a compatible...